Our AI-designed ad creatives are guaranteed to increase your ad conversion rate.
The success of an ad campaign relies on the effectiveness of the ad content. Aesthetically designed ad creatives can capture the attention of the viewers and influence their interest. An interesting ad creative can significantly improve the ad click-through rate (CTR).
Today, the advertising industry is saturated more than ever. Ad campaigns need to stand out to indulge potential customers.
Different advertising platforms cater to different audiences and have various design requirements. Advertisers are required to put in the extra work. They design hundreds of variations of ad creatives to appeal to their targeted audience. The variations allow a greater degree of personalization to attract relevant customers.
Advertisers perform A/B testing to compare the performance of different ad creative variations. Variations are shown to customers in a controlled environment to gather insights. It enables advertisers to make data-backed decisions and discard low-performing ad creatives. The remaining high-performing ad creatives promise a successful ad campaign and an increase in the business ROI.
Generating ad creative variations and running different tests requires extra time and human resources, making ad design boring, repetitive, and laborious.
For example, a client wants to design ads for five products, each with ten different size formats, a total of fifty banners. A designer would take hours to generate these banners, and the marketer would run various A/B tests to find their effectiveness.
AI can do it in seconds.
How Simple Ad Automation Differs From AI-Powered Advertisement
There are many automated ad creative design tools in the market. Although they are effective in generating ads, they cannot promise high conversion rates. Automation tools generate ad creatives based on fixed design schemes. They do not account for the relevance or context of the brand requirements. Nor do they consider market trends.
AI-powered marketing tools, on the other hand, learn from data and adapt automatically. The learning part is known as training the model.
Machine Learning (ML) and Deep Learning (DL) are two sub-categories of AI which deal with training models based on historical data. They have complex algorithms and techniques, each suited to carry out different tasks. The ultimate goal of ML and DL techniques is to predict the best possible outcome for a given input data.
The marketing industry is generating ad data in large numbers. Modern AI models can effectively use this data to design high-quality ad content at scale. Once trained, a robust AI model can consider brand themes and requirements to design dynamic ad creatives. As it learns from historical data, AI can reduce the effort required to test the ad designs.
Let us see how our AI-powered ad framework designs high-converting ad creatives at scale.

Collecting High-Quality Ad Creatives
The world is producing ginormous amounts of data every day. Companies can now derive real business value by making data-backed decisions--only if they know how to use it.
“Information is the oil of the 21st century, and analytics is the combustion engine.” – Peter Sondergaard, senior vice president, Gartner Research.
The effectiveness of an AI model relies on the quality of the data it trains on. Incorrect, inconsistent, biased, and noisy data can lead to unreliable and non-robust AI models.
Our dynamic AI engine feeds on millions of curated ad creatives collected through the Google Display Network. Our team regularly collects high-converting ad creatives from various industries.
Marketers and advertisers who work with us can also choose to connect their cross-channel ad accounts with our AI engine. We gather high-performing ad creatives from their previous successful campaigns. These ad creatives are usually targeted towards specific customers, which allows our AI engine to learn more about audience segmentation patterns. It helps our AI understand which ad creatives have a better chance of success.
Understanding the audience and their preferences are vital for us. Our partnership with marketers and advertisers allows us to collect consumer demographic information via their Facebook, Instagram, and Google advertising accounts. We also acquire relevant demographical and statistical insights about the audience using Google Analytics to improve the quality of our data.
We collect all of this information to optimize ad creatives for wide-ranging audiences. It allows our AI engine to develop personalized and high-converting ad creatives based on gender, age, location, and other digital preferences for advertising platforms of Facebook, Instagram, LinkedIn, Google, and Bing.
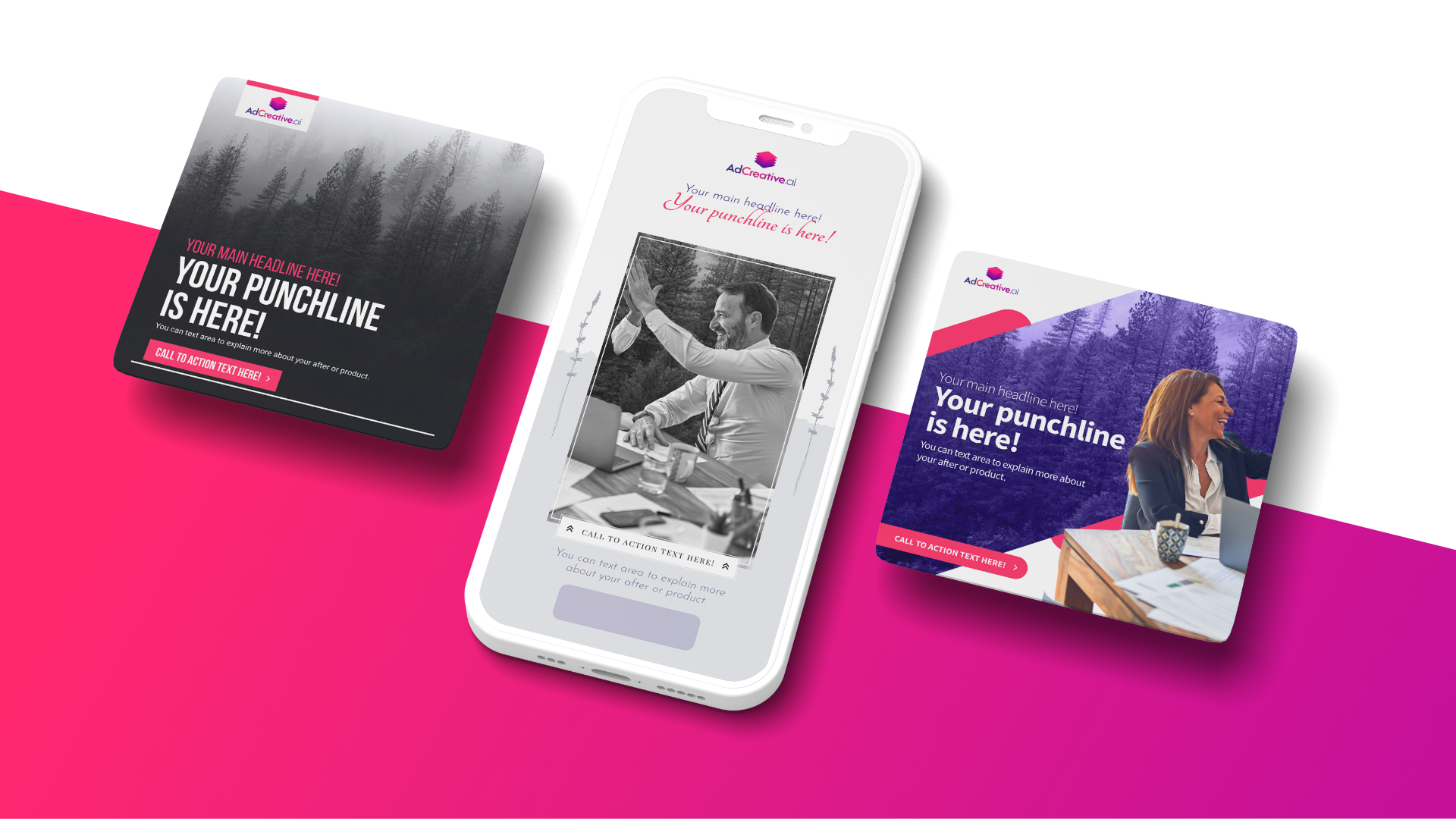
Data Features Needed To Design Dynamic Ad Creatives
Ad creatives consist of six major components: company logo, brand colors, banner size, ad copy text, background image, and product image.
We train our ML model on historically high-converting ad creatives. The model extracts and learns each of these features from the ad creatives.
Machine Learning processes different data types differently. Our ML model deals with visual data mostly. This data is in the form of the background image, product image, and logo. It also segments ad copy text from the ad creative. Our AI utilizes brand colors and banner size while generating ad creatives.
The goal of any ML model is to learn the patterns in the historical data and identify similar patterns in the unseen data.
“If somebody tortures the data enough (open or not), it will confess anything.” – Paolo Magrassi, former vice president, research director, Gartner.
When the users generate ad creatives using our AI engine, they provide all six input data features. The AI identifies patterns in the given data based on the historical ad creatives used in training. Once identified, the AI generates relevant designs which are similar to the ones it learned in training.
Ensuring Quality by Following the AI Principles
Our Machine Learning architecture follows the AI principles of transparency, robustness, explainability, scalability, and fairness. We have precisely calibrated our data and ML model to follow industry-standard guidelines.
We ensure transparency by periodically publishing guides like these, allowing our users to understand our AI ecosystem. We use an industry-standard cloud platform to deploy our ML pipeline securely.
Using the cloud platform enables us to scale our model automatically. Users can generate 10, 100, or 10000 ad creatives without bandwidth, memory, or size limitations.
Our model eliminates human bias, preferences, and errors when generating ad creatives. The generation process relies entirely on historical data belonging to all major industries. Our users can be sure that the generated designs have proven conversion rates.
However, AI systems are prone to uncertainties. As part of the commitment to AI transparency, our data-backed AI engine guarantees far fewer failures compared to manually designed ad creatives.
“Errors using inadequate data are much less than those using no data at all.” – Charles Babbage, mathematician, engineer, inventor, and philosopher.
AdCreative.AI's ML Architecture
Ad creatives are aesthetically designed images with various components. Only a powerful AI model can process these millions of ad creatives to find patterns.
Beware, technical jargon ahead!
Convolutional Neural Network (CNN or ConvNet) is a powerful Deep Learning technique that drives the core of our AI advertisement framework. It can recognize different objects, learn complex patterns, and works well with RGB images.
Our proprietary CNN model identifies and learns about all assets in the ad creatives. These are background images, product images, company logos, and ad copy text.
A neural network consists of interconnected layers. A typical CNN contains three types of layers: convolutional layer, pooling layer, fully connected layer. Convolutional layers and pooling layers are used in combination one after the other. The fully connected layer is used at the end of the network to make the final decisions. Together the layers form a hierarchy within the network.
Each convolutional layer performs a pixel-level analysis and learns the information stored within the ad creatives. The layers at the start identify high-level patterns like edges and curves. The layers at the end of the network identify complete objects like logo, product image, background, etc.
CNN is computationally demanding, and since our training data consists of millions of ad creatives, we need a compression mechanism to speed up the training process. Pooling layers reduce the data and the overall complexity of the network. The important information remains intact and the computations are kept manageable.
The fully connected layer identifies all the major components of the ad creatives and makes the final decision. The AI also learns the placement position for each ad creative component. The only thing left now is to generate beautiful ad designs.
The users input the ad creative assets into the AI engine. The trained AI model recognizes each of the components and generates possible layouts. Since the banner sizes can be different, the system meticulously checks for alignment and overlapping issues. The AI generates seamless and eye-catching designs and fits all the ad components in their respective positions.
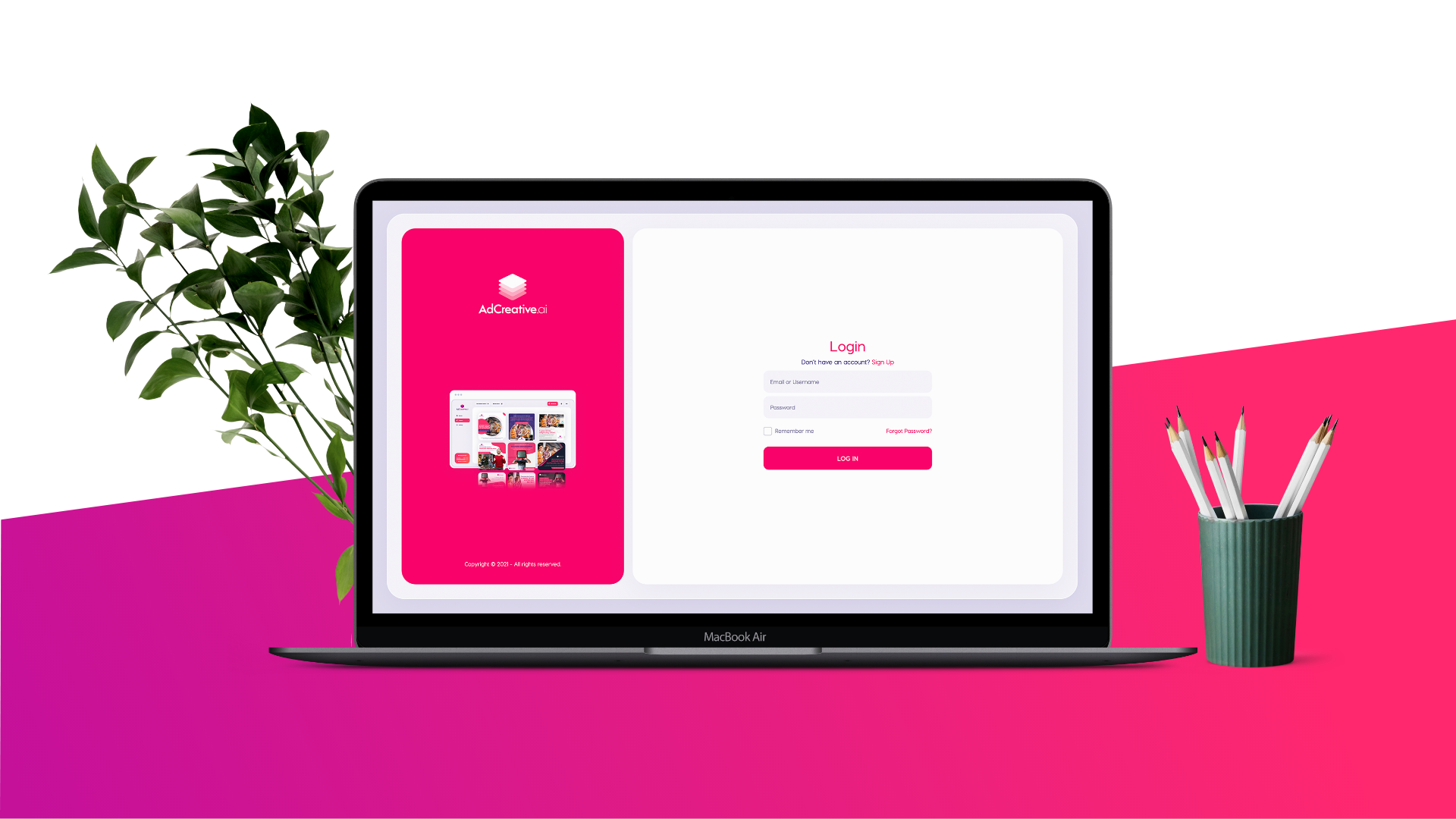
Concluding Thoughts
Creative strategists employ various marketing and technological techniques to improve the efficiency of their campaigns. AI-powered marketing tools can effectively reduce the cost of laborious and repetitive tasks allowing designers to enjoy creative freedom. They can spend more time bringing their creative imagination to life.
AdCreative.ai's ML engine can effectively design automated ad creatives. The ad creative variations generated by our AI are potent in reducing ad fatigue by frequently refreshing the viewers' memory.
Our AI is actively learning and improving. We are continuously training our AI engine by collecting high-converting ad creatives and user analytics. This allows us to keep our system up-to-date with the industry and consumer trends. Using our data-backed technology enables marketers to run ad campaigns without any rigorous A/B testing, further reducing the overhead costs and improving ROI.
We strongly believe that integrating AI with marketing can yield profitable outcomes for businesses. If you want to improve your chances of running successful ad campaigns, then design your ad creatives using our AI-powered automated advertising platform.